Generative Artificial Intelligence (Generative AI) is causing a significant upheaval in the data analytics sector. One modern invention that has the potential to completely transform data analytics across sectors is generative artificial intelligence. It was just a matter of time until businesses began using generative artificial intelligence (GenAI), which has been making waves for the better part of 2023, to inform their decisions. If statistics are to be believed, 10% of the world’s data generation is anticipated to come from generative artificial intelligence by 2025, according to Gartner.
Using Large Language Models (LLMs), Generative AI for data analytics creates synthetic data, recognizes intricate patterns in data, helps find anomalies, and presents data in an approachable manner. In the process, decision-makers gain from the empowerment of stakeholders and the decreased demand for data scientists.Â
The Rise of Generative AI in Data Analytics
The next development in artificial intelligence is generative AI. It encompasses a range of modern techniques and models, including Generative Adversarial Networks (GANs), Variational Autoencoders (VAEs), and language models such as OpenAI’s GPT series. These models have demonstrated remarkable capabilities, from creating lifelike images to producing human-like text.
One of the key strengths of generative AI is its ability to synthesize data that mirrors real-world patterns. By training on vast datasets, these models learn the underlying structure and characteristics of the data, enabling them to generate new, plausible data points that conform to these patterns. This profound capability opens up a world of possibilities for artificial intelligence in analytics, particularly artificial intelligence in data analytics and artificial intelligence for data analytics.
1. Generative Adversarial Networks (GANs)
It’s important to note that generative AI is not a brand-new technology. It was introduced in the 1960s with chatbots. However, it wasn’t until 2014 that generative AI evolved into its current form. Ian Goodfellow and his colleagues introduced Generative Adversarial Networks (GANs), which have since become one of the most prominent techniques in generative AI.
GANs, a type of machine learning algorithm, frame the problem as a supervised learning task involving two sub-models: a generator and a discriminator. The generator creates new data samples, while the discriminator classifies these samples as real or fake within the given domain. Through iterative training, the generator improves its ability to produce realistic data, and the discriminator enhances its skill in distinguishing between real and fake data.
2. Variational Autoencoders (VAEs)
Another prominent technique in generative modeling is the Variational Autoencoder (VAE). Proposed by Diederik P. Kingma and Max Welling in 2013, VAEs use an encoder-decoder architecture, setting them apart from traditional autoencoders. The encoder transforms raw data into a probability distribution within a lower-dimensional latent space, while the decoder reconstructs this data back into its original form. VAEs are particularly effective for generating realistic human faces or synthetic data for training AI systems.
3. Transformer Architecture in Deep Learning
In addition to GANs and VAEs, various other generative AI models exist, such as recurrent neural networks (RNNs), diffusion models, foundational models, and transformer architectures. The transformer architecture, developed by researchers at Google, has been instrumental in creating large language models (LLMs) like Google BERT, OpenAI’s ChatGPT, and Google AlphaFold. This architecture has significantly advanced the capabilities of generative AI in processing and generating human-like text.
The Emergence of Large-Scale Language Models (LLMs)
Large-scale language models (LLMs) are leading the way in Generative AI and Data Analysis. These models, backed by artificial intelligence, have piqued the interest of experts across sectors. Here’s how.
1. Human-Like Text Generation: LLMs can create text that resembles human language. Their grasp of context and subtlety enables them to construct meaningful and contextually appropriate phrases.
2. Multilingual Capabilities: Language learning machines can effortlessly convert text between languages. These methods cut down language barriers in a variety of contexts, including social media posts.
3. Sentiment Analysis: By analyzing text, LLMs may identify emotions, views, and attitudes. This feature has several uses, including consumer feedback analysis and brand reputation management.
4. Code Generation: LLMs can also produce code snippets. Whether it’s Python or JavaScript, such models can help the developers by suggesting code segments.
5. Creative Writing: Letter-of-meaning specialists also engage in artistic endeavors. They are able to create unique material that surprises and delights, ranging from poetry to short fiction.
What distinguishes LLMs is their capacity to generalize information across fields. They learn from a variety of datasets and sources. This adaptability offers great potential for a variety of areas, including healthcare, finance, marketing, and research, among others.
Generative AI, powered by LLMs, is altering our interactions with technology. As these models mature, the influence on data analytics and management will be significant.
Generative AI in analytics is not limited to professionals. It’s an effective tool that enables a larger audience to study data, discover hidden treasures, and make educated judgments. Generative AI is changing the way organizations run by making insights more accessible to everyone.Â
Generative AI for Data Science
A fundamental change in content generation is represented by generative AI. Generative AI for data science generates unique material, in contrast to typical AI models that depend on predetermined parameters. It functions in the deep learning space and sets itself apart by being able to produce new data labels depending on the input.
-
Getting Over Cognitive Bottlenecks
Cognitive biases and bottlenecks restrict human ideation by nature. Our capacity to produce and test ideas at scale and high throughput is hampered by these limitations. Moreover, we are limited in our ability to understand the massive volume of data that Fortune 200 businesses typically consume due to our connection speed.
By avoiding human prejudices and providing other approaches to utilizing data, analytical artificial intelligence fills in these gaps. It develops and evaluates theories using all the data sources at its disposal to produce particular business insights and overall reports.
-
Asking Appropriate Questions
Asking the proper questions is another benefit of generative AI. Similar to using ChatGPT, the questions we ask determine the caliber of the insights. Platforms such as the Discovery platform are able to generate and analyze millions of hypotheses per minute by utilizing curated functions. Teams may now assess ideas, integrate them with domain expertise, and make qualified impacts thanks to this technology.
Benefits of Generative AI for Data Analytics
Generative AI offers a multitude of benefits for data analytics, revolutionizing how businesses and researchers approach data analysis. By using artificial intelligence in analytics, organizations can unlock new levels of efficiency, insight, and innovation.
-
Enhanced Data Augmentation
One of the primary advantages of using generative AI in data analytics is its ability to augment existing datasets. Generative models can produce realistic synthetic data that mirrors the patterns and characteristics of real-world data. This capability is particularly valuable when working with limited datasets or when needing to simulate various scenarios without the risk or expense of collecting additional data. For instance, in healthcare, generative AI can create synthetic patient data that helps in training more robust and accurate predictive models.
-
Improved Predictive Modeling
Generative AI significantly enhances predictive modeling by providing high-quality data for training. Models like GANs and VAEs can generate diverse data samples, helping to address class imbalances and improve the generalizability of predictive models. This results in more accurate forecasts and better decision-making capabilities. AI in data analytics thus ensures that businesses can anticipate market trends, customer behavior, and operational risks with greater confidence.
-
Advanced Anomaly Detection
Incorporating data analysis artificial intelligence techniques, generative AI models can effectively identify anomalies within datasets. By learning the normal patterns of data, these models can detect deviations that may indicate fraud, errors, or other significant events. This is especially useful in industries like finance, where early detection of irregularities can prevent substantial losses and improve compliance.
-
Increased Data Privacy
Generative AI can also contribute to enhanced data privacy. By generating synthetic data that retains the statistical properties of the original dataset, organizations can share and analyze data without compromising sensitive information. This approach is particularly beneficial in sectors with strict privacy regulations, such as healthcare and finance, allowing for the safe use and distribution of data while maintaining compliance with privacy laws.
-
Accelerated Insights and Innovation
The use of AI in data analytics accelerates the discovery of insights and fosters innovation. Generative AI models can uncover hidden patterns and relationships within large datasets that might be missed by traditional analytical methods. This leads to deeper understanding and novel insights that can drive innovation across various domains, from product development to strategic planning.
Related: Guide to AI Workflow Automation
Generative AI represents a powerful tool for data analytics, providing enhanced data augmentation, improved predictive modeling, advanced anomaly detection, enhanced data privacy, and accelerated insights and innovation. By integrating artificial intelligence in analytics, organizations can harness these benefits to gain a competitive edge and drive data-driven success.
Generative AI for Data Lifecycle Management
Data lifecycle management involves overseeing data from its creation or acquisition through to its disposal. This process includes several phases, and the steps can vary depending on the organization and type of data. Generative AI for data analysis can be applied at various stages to enhance this process:
1. Data Extraction
- Web Scraping
Large Language Models (LLMs) are excellent at web scraping, capable of extracting text, links, and images from web pages. They comprehend text meanings, identify patterns, and summarize information. The extracted data is then pre-processed for further analysis. Genetic algorithms enhance web scraping by optimizing parameters, handling dynamic content, bypassing anti-scraping measures, and adapting to changes in websites.Â
Tools like browser automation frameworks, proxy rotators, and even ChatGPT scraping setups are increasingly being used to extract structured insights from sources such as job boards, eCommerce listings, competitor content, and pricing databases.
- Schema Inference & Data Parsing
Generative AI can infer data schemas and parse unstructured or semi-structured data. By training on sample data, these models learn patterns and extract structured elements, transforming raw data into a structured format. Generative AI refines schema inference and data parsing by continually optimizing algorithms to accurately infer data structures and handle diverse data formats efficiently.
- Transactional Data Extraction
LLMs can extract data from articles, documents, and data marketplaces, saving it in appropriate formats within Enterprise Data Platforms. For example, they extract financial data from reports, summarize it, and generate code for export to formats like JSON. They also extract transactional data from invoices and receipts in various formats, including PDFs. Generative AI enhances this process by iteratively optimizing algorithms to capture transaction details accurately and efficiently.
2. Data Integration
- Schema Mapping and Transformation
Generative models generate mapping rules and transformations after being trained on source and destination data schemas. This simplifies data integration, ensuring schematic alignment and providing audit reference documents. Generative AI improves schema mapping and transformation by continually optimizing algorithms to accurately map data between different schemas and adapt to evolving data structures.
- Entity Resolution and Matching
Generative AI is used for entity resolution and matching, identifying and linking entities across diverse datasets. This process is enhanced by continually optimizing algorithms to accurately match entities, improving efficiency and adaptability to varying data quality and matching criteria.
- Data Unification and Deduplication
Generative models, trained on existing data, learn patterns to identify duplicate records and generate rules for merging similar records. This streamlines data integration by eliminating duplicates.
3. Data Transformation
- Data Cleansing
LLMs identify and correct anomalies within datasets, assist in standardizing formats and perform deduplication tasks. Generative AI enhances data cleansing by optimizing algorithms to detect and correct errors, remove duplicates, and standardize data formats, thereby improving data quality and processing efficiency.
- Data Mapping and Transformation
Generative AI models, trained on source and target data schemas, create mappings and transformation rules. LLMs generate code for tasks such as merging, formatting, or filtering data. For instance, LLMs can transform data across the medallion data flow pattern (Bronze, Silver, Gold), refining and aggregating it to generate reports on Sales, Marketing, and Supply Chain/Logistics. They also help data analysts validate hypotheses and generate framework code for data transformation rules.
4. Data Discovery and Exploration
- Data Profiling
Generative AI analyzes dataset content, structure, and metadata to generate descriptive summaries, statistics, and visual representations such as distribution charts. Data profiling is enhanced by data discovery tools that iteratively optimize algorithms to accurately summarize data characteristics and identify patterns, anomalies, and relationships.
- Data Clustering and Classification
Generative models examine features and relationships to identify groups or categories and segment datasets. This process is refined by continually optimizing algorithms to group similar data points accurately and assign them to relevant categories.
- Exploratory Data Visualization
Generative AI supports exploratory data visualization by generating diverse visual formats, helping users interactively explore patterns, trends, and relationships. It creates visual representations like network graphs or relationship maps to uncover data dependencies.
- Anomaly/Outlier Detection
Generative AI models assist in detecting anomalies or outliers in datasets, flagging potential issues for further investigation. This process is enhanced by continually optimizing algorithms to identify deviations from normal patterns accurately, improving detection sensitivity and accuracy.
- Conversational Interfaces
Generative AI creates conversational AI, and natural language interfaces for data discovery, interpreting user queries, retrieving relevant data, and providing insights conversationally.
5. Data Quality
- Data Quality Assessment
Generative AI analyzes data patterns and distributions to identify anomalies, outliers, and potential quality issues, flagging erroneous, incomplete, and missing data for cleaning.
- Data Preprocessing
Preprocessing operations like feature scaling and missing value imputation are automated by generative AI. In order to ensure data consistency and quality, it forecasts missing numbers and uses standardization procedures.
- Data Synthesis and Augmentation
Generative AI generates synthetic data points that mirror the patterns of the original dataset, enhancing data for further exploration and hypothesis validation.
6. Data Orchestration
- Workflow Generation and Documentation
Generative models, trained on historical data and workflow patterns, can automatically generate workflow templates that capture data dependencies, task sequences, and operational procedures, ensuring efficient and auditable workflows.
- Task Scheduling Optimization
Generative AI assists in optimal task scheduling within data orchestration workflows by analyzing dependencies, resource constraints, and historical performance data to recommend efficient task execution sequences, minimizing resource bottlenecks, and ensuring timely data processing.
- Debugging and Error Handling
Generative models analyze error logs and historical data to identify common errors and generate recommendations for handling and recovering from failures. LLMs development can inspect and debug pipelines to ensure smooth data flow.
- Data Quality Validation and Anomaly Detection
Generative AI learns patterns and identifies potential data quality issues, flagging missing values, inconsistencies, and outliers during data pipeline monitoring. Anomalies are isolated, redacted, and archived to maintain data integrity.
- Automated Data Governance
Generative models assist in metadata capture, data lineage, and business rules enforcement, recommending data classification, access controls, and privacy compliance measures to ensure regulatory adherence and enforce organizational policies.
- Data Pipeline Optimization
By analyzing historical data, resource constraints, and pipeline performance, generative models suggest optimizations such as reordering steps, parallelization, and alternative processing techniques to improve efficiency and scalability.
7. Data Migration
Data migration involves moving data from one system or platform to another, requiring careful planning and execution. Generative AI streamlines this complex task:
- Data Domain Documentation
Generative AI assists in documenting data domains by analyzing datasets to discover data mappings, relationships, and semantics. This documentation is crucial for legacy systems where knowledge may be limited, ensuring a smooth migration process.
- Migration Rationalization
Generative models perform log analysis to identify usage patterns and generate reports comparing active and obsolete datasets. This helps optimize data migration strategies, focusing efforts on relevant data to achieve efficiency gains.
- Data Quality and Error Handling
Generative AI automates data quality assessment during migration by analyzing error logs to identify anomalies and inconsistencies and recommending error-handling strategies to ensure data integrity.
- Post-Migration Validation
After migration, LLMs and generative AI validate data consistency by summarizing and comparing datasets between the legacy and new platforms, ensuring data remains accurate and usable.
- Performance Optimization
Generative models analyze historical performance data and resource utilization patterns, recommending optimal configurations and strategies such as adjusting parallelism and fine-tuning resource allocation to enhance performance during migration.
Generative AI Tools for Data Analysis 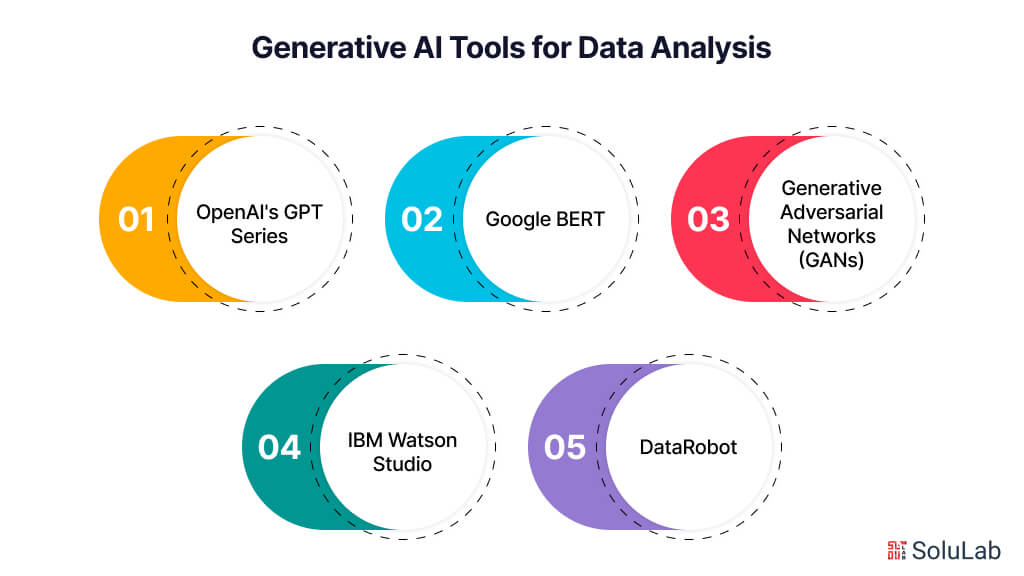
Generative AI tools are revolutionizing data analysis by providing advanced capabilities to generate, analyze, and interpret large datasets with remarkable accuracy and efficiency. Key tools include:
1. OpenAI’s GPT Series
OpenAI’s GPT models, including GPT-4, excel in natural language understanding and generation. They are used for:
- Text Analysis and Summarization: Extracting insights and generating concise summaries from large text datasets.
- Data Transformation: Converting unstructured data into structured formats.
- Predictive Modeling: Supporting predictive analytics with natural language explanations.
2. Google BERT
Google’s BERT model effectively understands the context of words in text data. It’s useful for:
- Text Classification: Categorizing text data into meaningful groups.
- Entity Recognition: Identifying and classifying entities within text.
- Sentiment Analysis: Gauging sentiment from textual data.
3. Generative Adversarial Networks (GANs)
A generator and discriminator collaborate to generate realistic data in GANs. They are used for:
- Image and Video Synthesis: Creating realistic images and videos.
- Data Imputation: Fill in missing data points.
- Synthetic Data Generation: Producing datasets that mimic real-world data.
4. IBM Watson Studio
IBM Watson Studio offers tools for building and deploying AI models, with features like:
- AutoAI: Automated model building and tuning.
- NLP Tools: Analyzing text data and generating reports.
- Data Refinery: Cleansing and transforming data.
5. DataRobot
DataRobot automates the process of building and deploying machine learning models, enhancing data analysis with:
- Automated Feature Engineering: Creating relevant features from raw data.
- Model Optimization: Improving model performance through automated tuning.
- Predictive Insights: Generating actionable insights from data.
These tools enable efficient, accurate, and insightful data analysis, helping organizations uncover patterns and make data-driven decisions confidently.
Use Cases for Generative AI in Data Analytics
Generative AI in analytics is transforming by providing advanced tools and methods for interpreting, analyzing, and generating data. These AI-driven approaches enhance various aspects of data analytics, making it possible to uncover deeper insights and automate complex tasks. Here are some key use cases:
1. Data Augmentation
Generative AI can create synthetic data that mirrors the characteristics of real-world data, thereby augmenting training datasets. This is particularly useful for improving the performance of machine learning models when original data is scarce or imbalanced. For instance, Generative Adversarial Networks (GANs) can generate additional images or text samples, enhancing the robustness of predictive models.
2. Anomaly Detection
Generative AI for data interpretation excels at detecting anomalies by learning the normal patterns in data and flagging deviations. Variational Autoencoders (VAEs) and GANs are commonly used to identify outliers in datasets such as transaction records, network logs, and sensor data, helping businesses detect fraud, network intrusions, or equipment failures.
Related: Top Generative AI Use Cases
3. Data Imputation
Artificial intelligence in analytics can effectively handle missing data through imputation. Generative AI models, such as VAEs, can predict and fill in missing values by understanding the data distribution. This improves the quality and completeness of datasets, ensuring more accurate analysis and decision-making.
4. Predictive Modeling
Generative AI enhances predictive analytics by creating models that can forecast future trends based on historical data. For example, AI for data analysis can generate scenarios and simulate outcomes, providing valuable insights for financial forecasting, demand planning, and risk assessment. Language models like OpenAI’s GPT can generate natural language reports summarizing predictive insights.
5. Data Synthesis
Generative AI can synthesize data that mimics real-world datasets, providing a valuable resource for testing and validating new algorithms and systems. This is especially beneficial in fields like healthcare and finance, where privacy concerns may restrict access to real data. Synthetic data allows for rigorous testing without compromising sensitive information.
6. Automated Reporting
Data analysis artificial intelligence can automate the generation of business reports by interpreting data and creating narratives. Tools like OpenAI’s GPT-4 can analyze datasets, identify key trends, and generate written summaries and visualizations. This streamlines the reporting process and ensures that stakeholders receive timely and accurate insights.
7. Data Integration
Generative AI facilitates data integration by automating the mapping and transformation of data from diverse sources. It can infer schemas, detect relationships, and generate transformation rules, simplifying the process of consolidating data into a unified format. This ensures consistency and accuracy across integrated datasets.
8. Customer Insights
AI for data analysis can generate insights into customer behavior by analyzing purchase patterns, feedback, and interaction data. Generative models can create detailed customer profiles and predict future behaviors, enabling personalized marketing strategies and improved customer experiences.
9. Scenario Simulation
Generative AI can simulate various scenarios to help organizations plan and manage risks. By generating possible future states based on current data, businesses can assess the impact of different strategies and make informed decisions. This is particularly useful in areas such as financial risk management, supply chain logistics, and strategic planning.
10. Data Visualization
Generative AI supports exploratory data analysis by generating diverse visualizations that help users identify patterns, trends, and relationships within datasets. It can create visual formats like network graphs or heatmaps, making complex data more accessible and interpretable.
These use cases demonstrate the profound impact of generative AI on data analytics. By leveraging artificial intelligence for data interpretation and analysis, organizations can unlock new levels of insight, efficiency, and accuracy in their data-driven endeavors.
Final Words
In conclusion, the integration of generative AI into data analysis and modeling represents a significant advancement in the field of artificial intelligence. By using the power of generative models like GANs, VAEs, and language models, organizations can unlock new possibilities for interpreting, synthesizing, and deriving insights from complex datasets. From data augmentation and anomaly detection to predictive modeling and automated reporting, generative AI offers a versatile toolkit for addressing various challenges in data analysis. As businesses continue to embrace data-driven decision-making, the adoption of generative AI technologies promises to drive innovation and efficiency across industries.
At SoluLab, we understand the transformative potential of generative AI in data analytics. As a leading AI development company, we specialize in utilizing advanced technologies to deliver customized solutions tailored to our clients’ specific needs. Whether you’re looking to enhance your data analysis capabilities, develop predictive models, or integrate generative AI into your workflow, our team of experts is here to help. Contact us today to explore how SoluLab can empower your organization with the latest advancements in artificial intelligence.
FAQs
1. What is generative AI, and how does it differ from other AI approaches?
Generative AI refers to a class of artificial intelligence techniques that focus on generating new data samples rather than simply analyzing existing data. Unlike traditional AI models that are primarily used for classification or prediction tasks, generative AI models like GANs and VAEs can create new data instances that closely resemble real-world data distributions.
2. How can generative AI benefit data analysis and modeling?
Generative AI offers several benefits for data analysis and modeling, including data annotation, anomaly detection, and predictive modeling. By generating synthetic data, detecting outliers, and forecasting future trends, generative AI enhances the depth and accuracy of data-driven insights, enabling organizations to make more informed decisions.
3. What are some practical applications of generative AI in real-world scenarios?
Generative AI has a wide range of applications across various industries. For example, in healthcare, it can be used to generate synthetic medical images for training diagnostic algorithms. In finance, it can help detect fraudulent transactions by generating synthetic data for anomaly detection. Additionally, in creative fields like art and design, generative AI can assist in creating novel and innovative content.
4. How do companies implement generative AI into their data analytics workflows?
Companies can implement generative AI into their data analytics workflows by leveraging pre-trained models or developing custom solutions tailored to their specific needs. This may involve training generative models on their own datasets, integrating them into existing analytics pipelines, and fine-tuning them to achieve desired outcomes.
5. What are the potential challenges and limitations of using generative AI in data analysis?
While generative AI offers numerous benefits, it also poses certain challenges and limitations. One common challenge is ensuring the quality and diversity of generated data, as poorly trained models may produce unrealistic or biased samples. Additionally, ethical considerations surrounding data privacy and security must be carefully addressed when generating synthetic data for sensitive applications.