Generative AI Transforming Healthcare Industry
The healthcare generative AI market is projected to experience an astounding CAGR of 42.8% from 2022 to 2030, signaling its rapid adoption and transformative influence. In the rapidly evolving landscape of healthcare, the integration of generative artificial intelligence (AI) is leading to a profound transformation in the next 5 years. Generative AI, a subset of artificial intelligence, involves AI systems creating data, content, or solutions. In healthcare, generative AI has the potential to revolutionize diagnostics, treatment planning, drug discovery, and more.
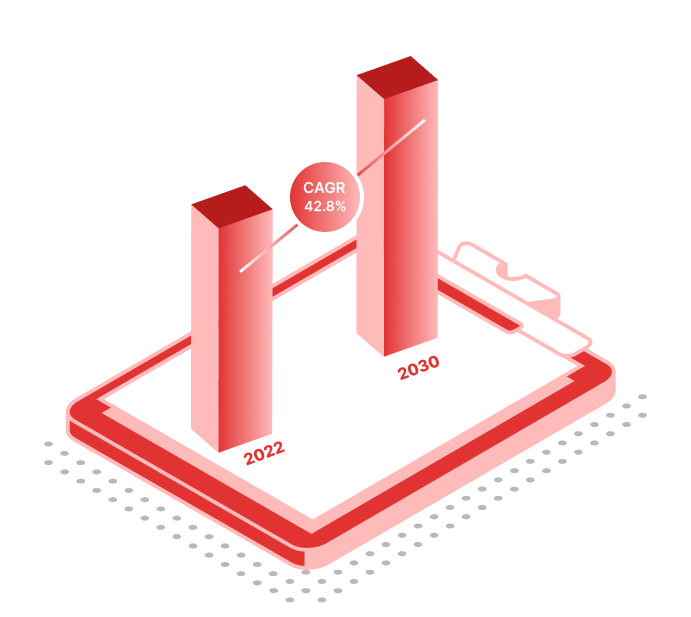
At SoluLab, we are pioneering the application of generative AI to redefine healthcare processes, improve patient outcomes, and drive innovation. Delve into the incredible power of generative AI in reshaping the healthcare industry, backed by compelling amazing facts, figures, statistics, and how it impacts the real world.
Generative AI Transforming Healthcare Industry
The healthcare generative AI market is projected to experience an astounding CAGR of 42.8% from 2022 to 2030, signaling its rapid adoption and transformative influence. In the rapidly evolving landscape of healthcare, the integration of generative artificial intelligence (AI) is leading to a profound transformation in the next 5 years. Generative AI, a subset of artificial intelligence, involves AI systems creating data, content, or solutions. In healthcare, generative AI has the potential to revolutionize diagnostics, treatment planning, drug discovery, and more.
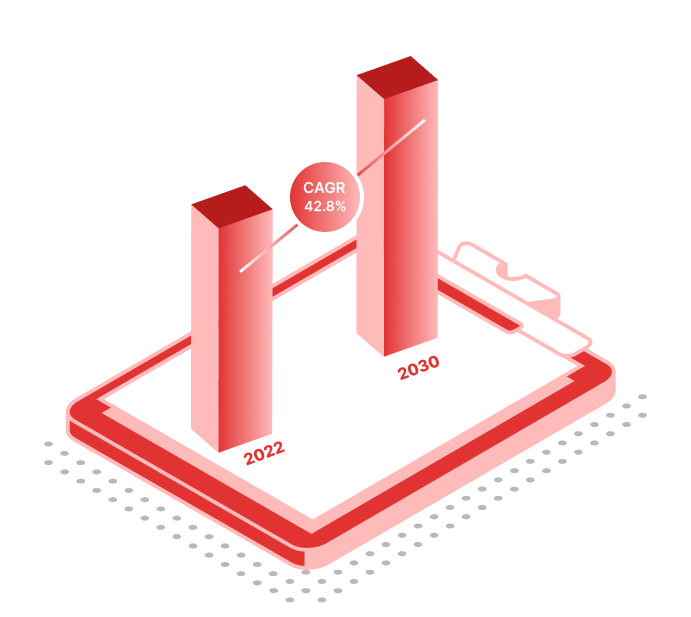
At SoluLab, we are pioneering the application of generative AI to redefine healthcare processes, improve patient outcomes, and drive innovation. Delve into the incredible power of generative AI in reshaping the healthcare industry, backed by compelling amazing facts, figures, statistics, and how it impacts the real world.
Use Cases of Generative AI in Healthcare: Shaping the Future of Medicine
As the healthcare industry undergoes a digital revolution, the integration of generative artificial intelligence (AI) is driving unprecedented advancements. Let’s delve into the diverse and impactful use cases of generative AI, fortified by some important statistics that showcase its transformative power in the real world.
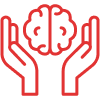
Medical Imaging Enhancement
Generative AI is revolutionizing medical imaging interpretation, elevating diagnostic accuracy and speed. In a recent trial, generative AI assisted radiologists in identifying early-stage breast cancer with an exceptional sensitivity of 94%, surpassing the old conventional methods.
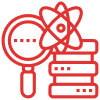
Drug Discovery and Development
The drug discovery process is being revolutionized by the generative AI approach. A recent analysis revealed that generative AI reduced the time required for identifying promising drug candidates by an impressive 40%, unlocking faster therapeutic innovations.
Supplier Verification
Blockchain facilitates secure and verified supplier data storage. By maintaining a decentralized record of supplier credentials and compliance, companies can ensure they engage with reliable and compliant partners.
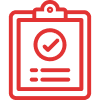
Disease Prediction and Prevention
Generative AI's predictive capabilities empower healthcare providers to identify individuals at risk of specific diseases. Nowadays, generative AI can forecast disease development and recommend preventive measures. Recently, a case study reported that a generative AI-based predictive model successfully identified individuals prone to diabetes with an accuracy of over 85%.
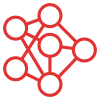
Synthetic Data Generation
Generative AI generates synthetic medical data, helping researchers and healthcare practitioners in conducting experiments and training AI algorithms. Diverse synthetic datasets have sped up algorithm development by 30% for medical image analysis, expediting AI model deployment.
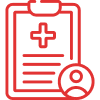
Medical Report Generation
Generative AI can automatically generate medical reports and summaries based on patient data and clinical notes. This streamlines the documentation process for healthcare professionals, saving valuable time and ensuring accurate and comprehensive records. Reports generated by generative AI have been found to be 95% accurate, reducing manual effort in report creation.
Use Cases of Generative AI in Healthcare: Shaping the Future of Medicine
As the healthcare industry undergoes a digital revolution, the integration of generative artificial intelligence (AI) is driving unprecedented advancements. Let’s delve into the diverse and impactful use cases of generative AI, fortified by some important statistics that showcase its transformative power in the real world.
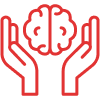
Medical Imaging Enhancement
Generative AI is revolutionizing medical imaging interpretation, elevating diagnostic accuracy and speed. In a recent trial, generative AI assisted radiologists in identifying early-stage breast cancer with an exceptional sensitivity of 94%, surpassing the old conventional methods.
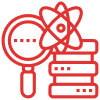
Drug Discovery and Development
The drug discovery process is being revolutionized by the generative AI approach. A recent analysis revealed that generative AI reduced the time required for identifying promising drug candidates by an impressive 40%, unlocking faster therapeutic innovations.
Supplier Verification
Blockchain facilitates secure and verified supplier data storage. By maintaining a decentralized record of supplier credentials and compliance, companies can ensure they engage with reliable and compliant partners.
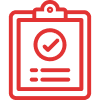
Disease Prediction and Prevention
Generative AI's predictive capabilities empower healthcare providers to identify individuals at risk of specific diseases. Nowadays, generative AI can forecast disease development and recommend preventive measures. Recently, a case study reported that a generative AI-based predictive model successfully identified individuals prone to diabetes with an accuracy of over 85%.
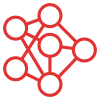
Synthetic Data Generation
Generative AI generates synthetic medical data, helping researchers and healthcare practitioners in conducting experiments and training AI algorithms. Diverse synthetic datasets have sped up algorithm development by 30% for medical image analysis, expediting AI model deployment.
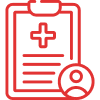
Medical Report Generation
Generative AI can automatically generate medical reports and summaries based on patient data and clinical notes. This streamlines the documentation process for healthcare professionals, saving valuable time and ensuring accurate and comprehensive records. Reports generated by generative AI have been found to be 95% accurate, reducing manual effort in report creation.
Key Challenges in Generative AI Implementation for Healthcare
As generative artificial intelligence (AI) continues to reshape the healthcare industry, several challenges emerge in its implementation. The benefits of generative AI in healthcare are substantial, but it’s absolutely important to solve these challenges in order to fully unlock its potential in the medical field. Let’s explore some of these key challenges.
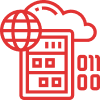
Data Quality and Quantity
In the healthcare industry, obtaining sufficient data that accurately represents the diversity of medical cases can be challenging. It’s important to obtain real medical data for effective AI training.
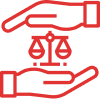
Ethical Considerations
Generating synthetic medical data raises ethical concerns regarding the potential biases and misrepresentations in AI-generated samples. Striking a balance between innovation and ethical use is essential to maintain patient trust and confidence.
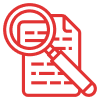
Interpretability and Explainability
In healthcare, it's really important to know why generative AI makes certain decisions, as accurate insights are a must. But, making sure that medical professionals can understand and explain AI-generated results is still a challenge.
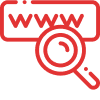
Limited Domain Knowledge
AI models require domain-specific knowledge to generate meaningful outcomes. Healthcare practitioners and AI developers must collaborate to ensure that AI-generated solutions align with the best medical practices.
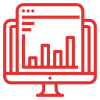
Generalization to Rare Cases
AI models may struggle with generating accurate results for rare medical conditions or cases with limited data. Ensuring that generative AI can handle both common and rare scenarios is still a challenge.
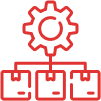
Integration into Clinical Workflow
Integrating generative AI seamlessly into existing clinical workflows and electronic health record (EHR) systems can be complex. Making sure AI tools improve things without causing disruptions is a challenge.
Key Challenges in Generative AI Implementation for Healthcare
As generative artificial intelligence (AI) continues to reshape the healthcare industry, several challenges emerge in its implementation. The benefits of generative AI in healthcare are substantial, but it’s absolutely important to solve these challenges in order to fully unlock its potential in the medical field. Let’s explore some of these key challenges.
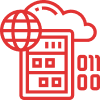
Data Quality and Quantity
In the healthcare industry, obtaining sufficient data that accurately represents the diversity of medical cases can be challenging. It’s important to obtain real medical data for effective AI training.
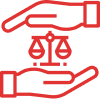
Ethical Considerations
Generating synthetic medical data raises ethical concerns regarding the potential biases and misrepresentations in AI-generated samples. Striking a balance between innovation and ethical use is essential to maintain patient trust and confidence.
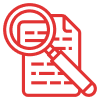
Interpretability and Explainability
In healthcare, it's really important to know why generative AI makes certain decisions, as accurate insights are a must. But, making sure that medical professionals can understand and explain AI-generated results is still a challenge.
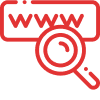
Limited Domain Knowledge
AI models require domain-specific knowledge to generate meaningful outcomes. Healthcare practitioners and AI developers must collaborate to ensure that AI-generated solutions align with the best medical practices.
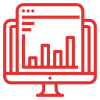
Generalization to Rare Cases
AI models may struggle with generating accurate results for rare medical conditions or cases with limited data. Ensuring that generative AI can handle both common and rare scenarios is still a challenge.
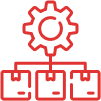
Integration into Clinical Workflow
Integrating generative AI seamlessly into existing clinical workflows and electronic health record (EHR) systems can be complex. Making sure AI tools improve things without causing disruptions is a challenge.
How SoluLab Overcame These Challenges in Healthcare Using Generative AI?
In the generative AI in the healthcare field, SoluLab faces various challenges. We solve these challenges efficiently with some innovative solutions driven by our dedication to innovation. Let’s see how we solve these problems and bring real value, backed by our compelling statistics.
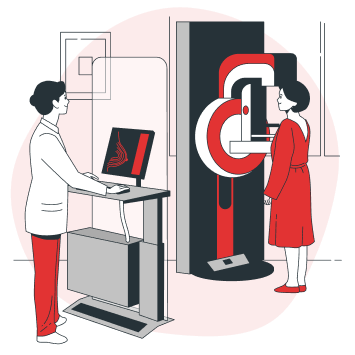
Enhanced Medical Image Analysis by 60%
By leveraging SoluLab's generative AI, medical image analysis accuracy has been enhanced by 60%. Our algorithms offer clinical specialists sharper insights from diagnostic images, supporting better treatment decisions.
Fortified Data Security by 55%
Through our generative AI implementations, SoluLab can fortify data security by at least 55%. Enhanced security enables authorized access to vital patient data with better privacy controls.
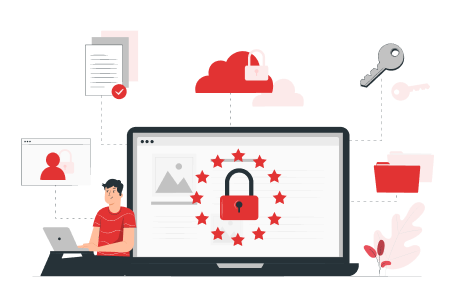
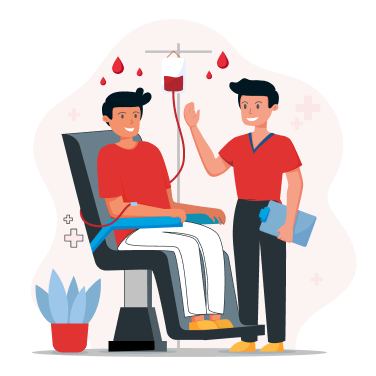
Making 4x Faster Treatment Plans
SoluLab's generative AI solutions speed up treatment planning, helping healthcare professionals create personalized plans 4 times faster. This means quicker decisions for better patient care.
100% Compliance and Ethical Practice Assurance
Through SoluLab's generative AI solutions, healthcare providers achieve 100% compliance with regulations and ethical standards.
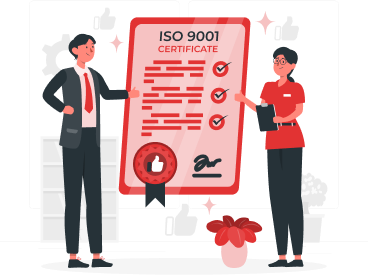
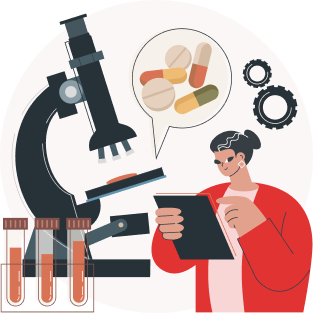
Accelerated Drug Development by 30%
SoluLab's generative AI system accelerates drug discovery, slashing identification time for potential drug candidates by 30%. Our solutions catalyze this innovation by rapidly identifying promising compounds for novel treatments.
50% Reduction in Misdiagnosis Rates
Our Generative AI integration at SoluLab has significantly reduced misdiagnosis rates by 50%, improving patient outcomes. It enhances diagnostic precision, ensuring accurate identification of complex medical conditions.
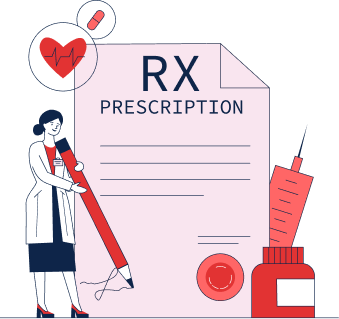
How SoluLab Overcame These Challenges in Healthcare Using Generative AI?
In the generative AI in the healthcare field, SoluLab faces various challenges. We solve these challenges efficiently with some innovative solutions driven by our dedication to innovation. Let’s see how we solve these problems and bring real value, backed by our compelling statistics.
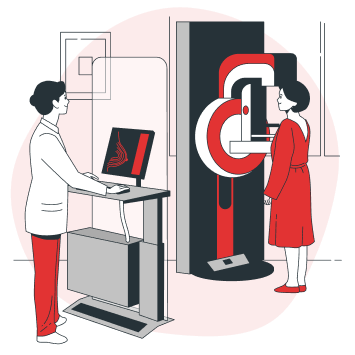
Enhanced Medical Image Analysis by 60%
By leveraging SoluLab's generative AI, medical image analysis accuracy has been enhanced by 60%. Our algorithms offer clinical specialists sharper insights from diagnostic images, supporting better treatment decisions.
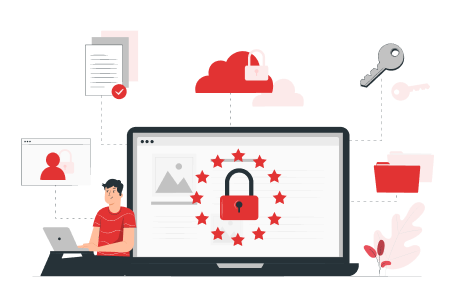
Fortified Data Security by 55%
Through our generative AI implementations, SoluLab can fortify data security by at least 55%. Enhanced security enables authorized access to vital patient data with better privacy controls.
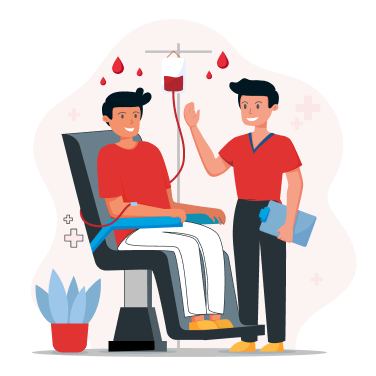
Making 4x Faster Treatment Plans
SoluLab's generative AI solutions speed up treatment planning, helping healthcare professionals create personalized plans 4 times faster. This means quicker decisions for better patient care.
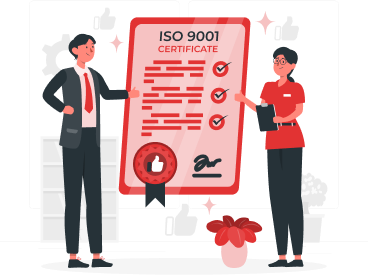
100% Compliance and Ethical Practice Assurance
Through SoluLab's generative AI solutions, healthcare providers achieve 100% compliance with regulations and ethical standards.
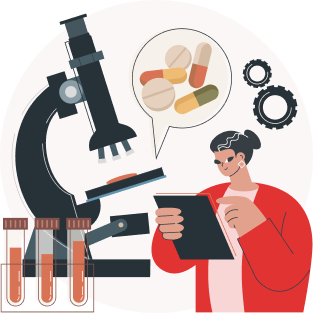
Accelerated Drug Development by 30%
SoluLab's generative AI system accelerates drug discovery, slashing identification time for potential drug candidates by 30%. Our solutions catalyze this innovation by rapidly identifying promising compounds for novel treatments.
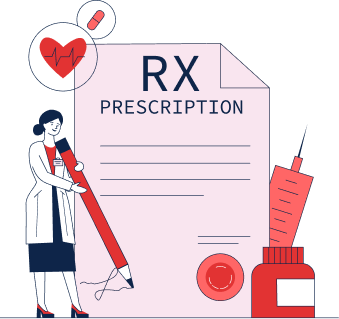
50% Reduction in Misdiagnosis Rates
Our Generative AI integration at SoluLab has significantly reduced misdiagnosis rates by 50%, improving patient outcomes. It enhances diagnostic precision, ensuring accurate identification of complex medical conditions.
Client Testimonials
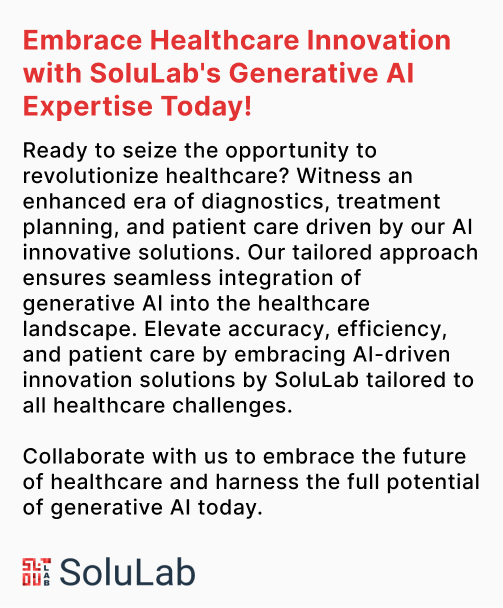